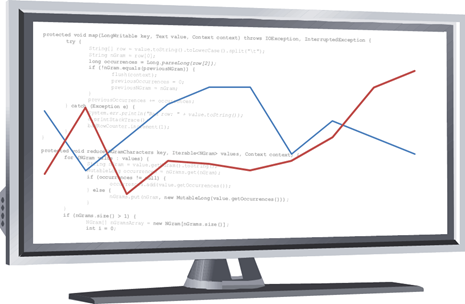
Today is all about being data-driven. And the first thing you need for being data-driven is metrics (or so called Key Performance Indicators – KPIs, if you like acronyms). So we all scramble to establish our metrics quickly, so that we can start being data-driven as soon as possible. And thus we often fall into a trap, because bad metrics can cause much more harm than being data-driven can cause good in the first place. There are plenty of excellent examples of bad metrics and their destructive power in the literature, but I would like to call out some examples from the area which is closest to my heart: software engineering.
Example 1: Number of bugs fixed
Today’s bug fix results:
Bob: 10 Iwona: 5 Steve: 3 Anna: 2 |
The e-mails had an immediate effect: everything just stopped working. Why? Well, we all felt pressured – probably more in front of each other than in front of anybody else. Everybody wanted to be high on that list for the sheer pleasure of being on top of a list. So we all automatically switched our behavior to optimize for the metrics we were presented with.
But everybody has limited resources and everything is a tradeoff, so optimizing one thing means sacrificing another. So we focused on fixing bugs as fast as we could. And since there were many bugs to choose from, we fixed the ones which allowed us to fix more in the finite time we had, such as “First letter in address label should be capitalized.” We skipped over the bugs which were really hard to fix, but of course those were the ones which were the most important.
Example 2: Code coverage
Here is a made up example, but in the spirit of what I observed. Let’s say we are testing the following function:
public static boolean extractFlag(Integer flags, Integer bit) {
if (flags == null) { throw new IllegalArgumentException(); } if (bit == null) { throw new IllegalArgumentException(); } return (flags.intValue() >>> bit.intValue()) % 2 > 0; }
Here is what a reasonable set of unit tests may have looked like before code coverage:
@Test
public void testExtractFlag1() { assertFalse(FlagUtils.extractFlag(2, 0)); } @Test public void testExtractFlag2() { assertTrue(FlagUtils.extractFlag(2, 1)); } @Test public void testExtractFlag3() { assertFalse(FlagUtils.extractFlag(Integer.MAX_VALUE, 31)); } @Test public void testExtractFlag4() { assertTrue(FlagUtils.extractFlag(-1, 31)); }
The above would have been pretty useful tests. They would have uncovered all of the following common mistakes on the last line of the function:
return (flags.intValue() / (1 << bit.intValue())) % 2 > 0;
return (flags.intValue() / bit.intValue()) % 2 > 0; return (flags.intValue() >> bit.intValue()) % 2 > 0;
But the coverage of these tests is pretty bad. They don’t cover all of the exception cases. So once the code coverage mandate was put into place, tests started morphing into something like this:
@Test(expected=IllegalArgumentException.class)
public void testExtractFlag1() { FlagUtils.extractFlag(null, 0); } @Test(expected=IllegalArgumentException.class) public void testExtractFlag2() { FlagUtils.extractFlag(0, null); } @Test public void testExtractFlag3() { FlagUtils.extractFlag(2, 1); } @Test public void testExtractFlag4() { FlagUtils.extractFlag(3, 1); }
Even though this particular example is made up, the general idea is exactly what happened: I literally saw hundreds of unit tests without a single assertion statement in them. But guess what, 100% coverage. Needless to say, these unit tests would not catch any of the common mistakes listed above.
Example 3: Lines of code
Examples of good metrics
- Number of customer complaints, bugs found by users, or bugs found in production
- Application efficiency (speed, resource consumption, etc.)
- System downtime
No comments:
Post a Comment